Top 5 Tips for Starting Your Modeling Career in 2025
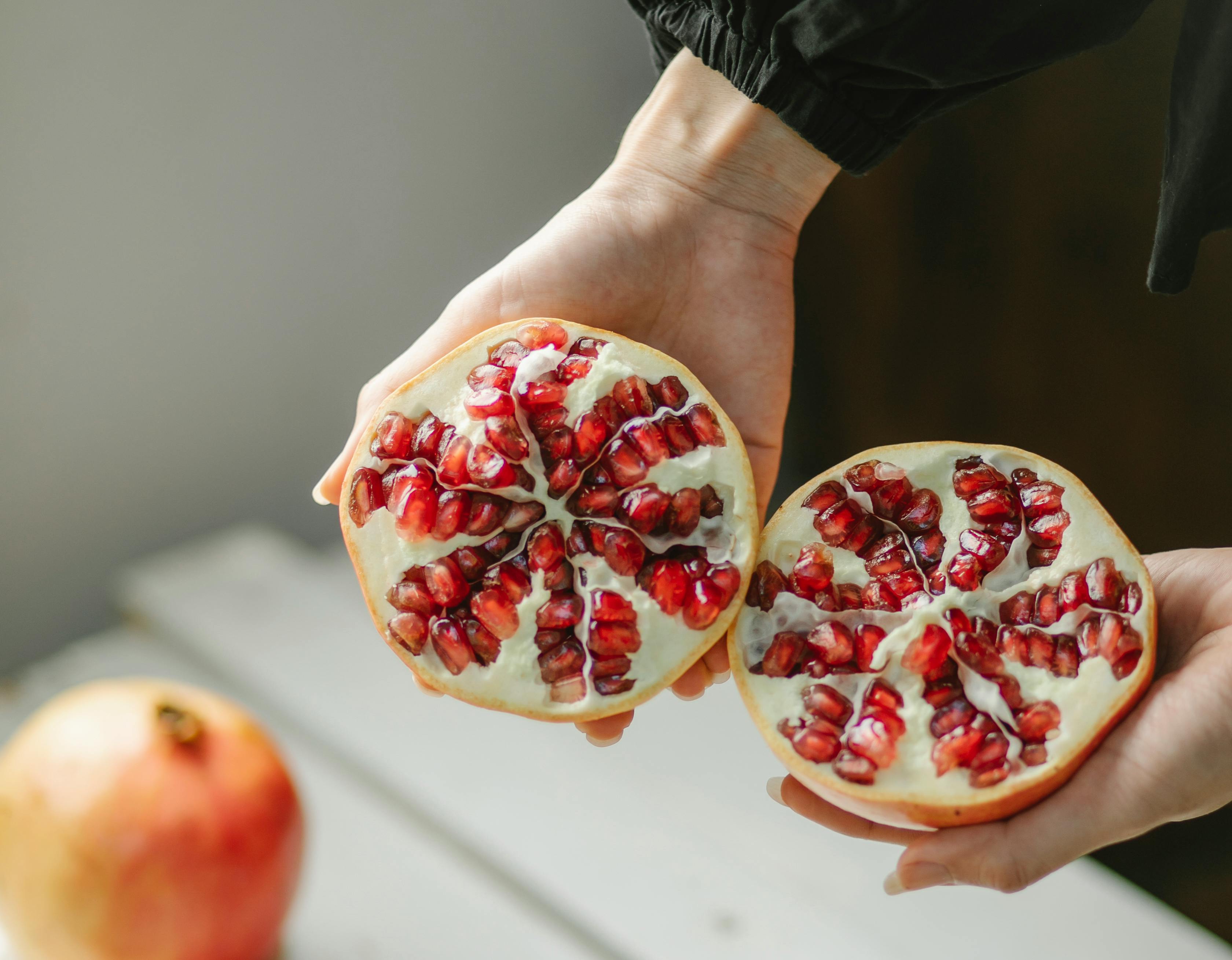
How to Start Modeling: Practical Tips for Success in 2025
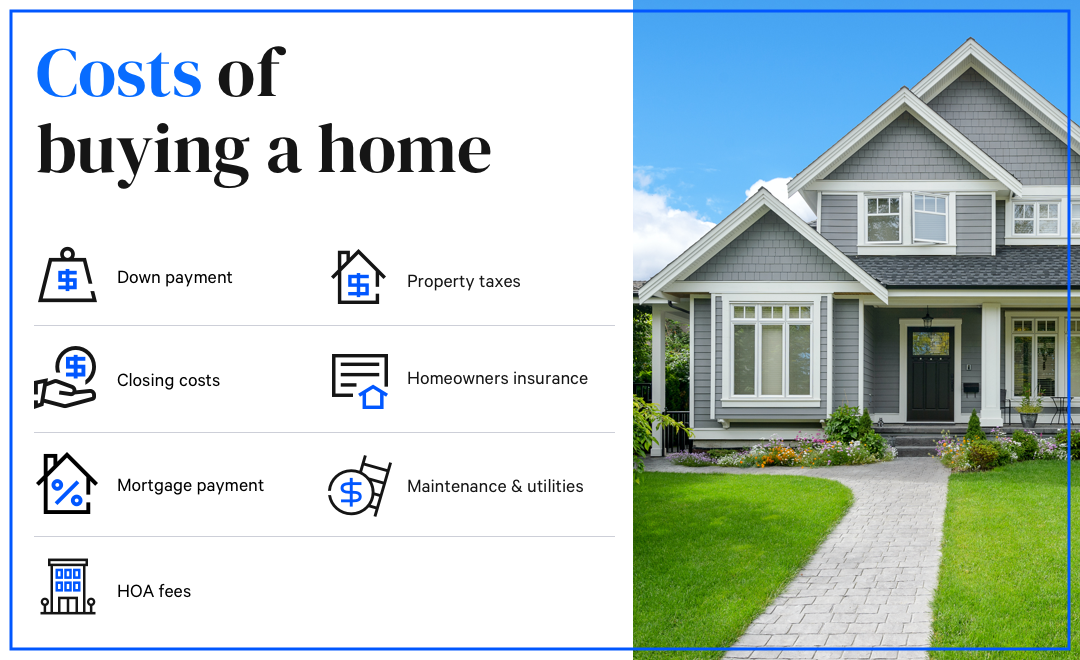
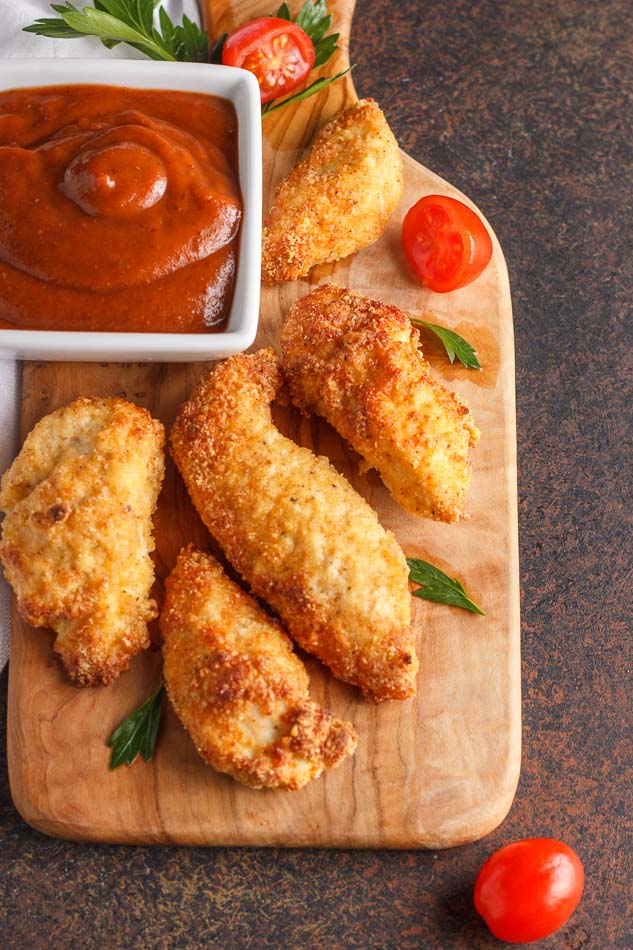
Understanding the Basics of Modeling Techniques
Modeling techniques are foundational to various fields, from data analysis to predictive modeling. They involve creating abstract representations of systems or processes to understand them better and predict future outcomes. Whether you are interested in statistical modeling or machine learning modeling, knowing the fundamentals is essential. For instance, principles of conceptual modeling allow you to outline the structure of a system, helping stakeholders understand the framework. To get started, familiarize yourself with definitions and categories like logical modeling, physical modeling, and dimensional modeling, which serve different purposes. Emphasizing clarity in model documentation is vital to building upon these techniques.
An Overview of Different Modeling Approaches
In the vast landscape of modeling, various approaches cater to unique needs. For beginner modelers, understanding types such as regression modeling, classification modeling, and time series modeling can shape your modeling journey. Regression modeling helps analyze relationships between datasets, while classification modeling sorts data into categories. Time series modeling, on the other hand, is essential for forecasting future values based on historical data. Each approach employs specific methodologies that enhance model performance and adaptation. This foundational knowledge enables you to select the appropriate modeling technique for your project objectives.
Common Challenges in Modeling
Starting your modeling career isn't without challenges. Common difficulties include accurately gathering data, dealing with model selection dilemmas, and overcoming obstacles during model evaluation. Effective data preparation and transformation techniques can alleviate some issues, as can thorough cross-validation methods to ensure model reliability. It’s not uncommon to encounter errors that necessitate iterative modeling and refinement. Emphasizing robust testing during model validation and employing best practices in modeling can help you navigate these hurdles successfully.
Steps to Develop a Successful Model
Building a successful model requires a systematic approach. It often begins with defining the problem and objectives clearly. Understanding the end goal determines the modeling framework you will use. Next, engage in model design to create a blueprint utilizing tools such as simulation modeling, causal modeling, or exploratory modeling methods. During the model training phase, ensure you select suitable data and parameters that yield insightful analytics post-development. Finally, it’s crucial to execute model deployment effectively, making applications such as operational modeling or real-time modeling comfortable for users.
Modeling Frameworks and Tools
Choosing the right modeling software and tools is crucial for seamless development. Software modeling tools can aid in product modeling, financial modeling, or even system dynamics modeling by providing tailored environments for creation and alteration. Familiarize yourself with collaborative modeling platforms that facilitate communication and inputs from team members. Incorporate advanced modeling techniques for scalability and robustness, allowing for optimal model performance over time.
Best Practices in Model Development
Adhering to best practices can significantly enhance the success and reliability of your models. This includes maintaining rigorous model documentation and following industry standards during the creation and testing phases. Ensure a user-centered modeling process, understanding your audience's needs while iterating based on feedback. Implementing a cycle of model optimization and validation increases accuracy and boosts end-user confidence in the model application. The simplification of processes through effective design modeling further enhances usability.
Exploring Advanced Modeling Techniques
As you grow in your modeling endeavors, exploring advanced techniques such as Bayesian modeling or hybrid modeling can lead to groundbreaking projects. Understanding structural equation modeling allows for deep insights into complex behavior patterns within your data sets. Moreover, engaging with artificial intelligence applications in modeling, like neural network modeling, offers avenues for innovative predictions and work efficiencies. Statistical analysis becomes integral here, guiding model evaluation and optimization based on significant metrics.
Real-World Applications of Modeling
Modeling's impact is widespread across industries, showcased through predictive analytics in finance, risk modeling in project management, and industry modeling in operations. Each sector applies tailored methodologies drawing upon best practices in modeling, leading to improved outcomes. Familiarizing yourself with real-world scenarios through case studies aids in recognizing how theoretical knowledge converts to concrete applications. Continuous exposure to challenges encountered by industry players is invaluable for developing skills applicable to your specific discipline.
Future Trends in Modeling
The landscape of modeling is continually evolving, with trends like cloud-based modeling and data governance shaping how models are developed and used. The rise of big data modeling underscores the significance of robust data infrastructure to support real-time analytics. Staying updated with advances in industry standards and ethics in modeling ensures that you implement responsible practices. As you anticipate emerging opportunities, maintaining awareness of innovations such as conversational agent modeling and automation will position you as a leader in your field.
Key Takeaways
- Understand modeling techniques and their basic principles.
- Choosing appropriate tools and frameworks is essential for development.
- Best practices must be adhered to for model reliability.
- An exploration of advanced techniques can enhance predictive capabilities.
- Stay informed about future developments within the modeling field.
FAQ
1. What are the main types of modeling techniques?
Modeling techniques vary significantly and include types such as statistical modeling, predictive modeling, and causal modeling. Each serves specific purposes ranging from data analysis to simulations, making it essential to understand their applications and implications in various fields.
2. How can I improve my model performance?
Improving model performance involves several strategies, including optimizing parameters through iterative modeling, utilizing cross-validation techniques, and ensuring robust model documentation. Implementing feedback and adapting based on model evaluation results are crucial for enhancing efficacy.
3. What are the benefits of collaborative modeling?
Collaborative modeling promotes teamwork and diverse input, enhancing model quality through shared insights. Engaging in collaborative platforms allows for effective communication and mutual corrective feedback, leading to more thoughtful model development and application.
4. How important is model validation?
Model validation ensures that the models produce reliable results before deployment. Rigorous testing helps identify potential flaws, improve trustworthiness, and confirm that the model meets intended purposes, which is crucial for avoiding decision-making based on inaccurate data.
5. Which modeling software should I use?
The choice of modeling software depends on your specific needs and industry. However, popular options include MATLAB for mathematical modeling, R for data analysis, and specialized modeling platforms like Simul8 or AnyLogic for simulation-based modeling, catering to various applications.